- Chimiométrie 2016 Challenge -
Indirect Quantitative Structure-Retention Relationship for Steroids Identification
Quantitative Structure–Retention Relationship (QSRR) is a very helpful method for the identification of unknown analytes by predicting their retention time, e.g. in liquid chromatography. More specifically, accurate retention time prediction constitutes an important support in the context of steroid identification, because of the many isomers that look similar in a mass spectrometer (i.e. identical m/z values).
Additionally, the indirect prediction of retention times using the linear solvent strength (LSS) parameters S and Log Kw has the great advantage of being applicable under any gradient conditions.
In the proposed dataset, experimental S and Log Kw values were estimated using Ultra High Pressure Liquid Chromatography (UHPLC) separations with two linear gradients (5-95% ACN + 0.1% FA) of 15 and 60 minutes respectively.
The aim of the study is to predict S and Log Kw using a multivariate model based on a series of molecular descriptors. The LSS theory is then applied to have accurate estimations of retention times for a 45 minutes gradient using those predicted S and Log Kw values .
The observations correspond to a database of reference steroid compounds. A series of molecular descriptors were calculated from their structures using the VolSurf+ software. By these means, a collection of 128 variables related to molecular shape, volume, polarisability, polar surface area, hydrophobic surface area, lipophilicity, molecular diffusion, and solubility, was generated automatically. These descriptors are conformation-independent since up to 50 conformers were considered for each structure.
The dataset includes 76 steroid compounds for calibration and 19 for validation.
Each molecule is characterised by 128 variables. Experimental Log Kw, S and retention time values are provided for the calibration set only.
The goal is to get the smallest RMSEP on the retention time predictions of the validation set for a 45 minutes gradient using LSS parameters.
Ideally, each individual relative error should not exceed 5% of the experimental retention time for both calibration and validation sets.
Excel spreadsheet
- Sheet 1 : Calibration set
Column A Compound names (Observations ID)
Column B Calibration labels
Columns C:DZ VolSurf descriptors (Independent variables)
Columns EA:EB Experimental LSS Parameters (Dependent variables - LogK and S)
- Sheet 2 : Calibration LSS RT Calculation
Column A Compound names (Observations ID)
Column B Calibration labels
Columns C:D Experimental LSS Parameters (Dependent variables - LogK and S)
Column J Retention time calculated by LSS model for predicted LogK and S
Column K Experimental Retention time
Column L Relative error in Retention time
Column A Compound names (Observations ID)
Column B Validation labels
Columns C:DZ VolSurf descriptors (Independent variables)
Columns EA:EB Insert predicted LSS Parameters here
- Sheet 4 : Validation LSS RT Calculation
Column A Compound names (Observations ID)
Column B Validation labels
Columns C:D Insert predicted LSS Parameters (LogK and S) here
Column J Retention time calculated by LSS model for predicted LogK and S
LSS equations
Automated calculations of tR from Log Kw and S are already included in the Excel spreadsheet
(Sheets 2 & 4, masked columns E:I).
(eq.1)
(eq. 2)
(eq.3)
Data can be downloaded at : Challenge.zip
Participants who wish to compete for prizes must submit their predictions (excel) of the validation set and their approach (doc or ppt) by 10 January 2016 to julien.boccard@unige.ch
The participants with the best results will be asked to present their approach during the conference.
Sponsored for the award of the best results by
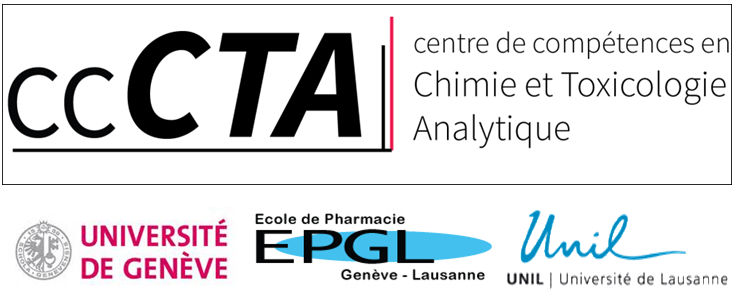